07 October 2024
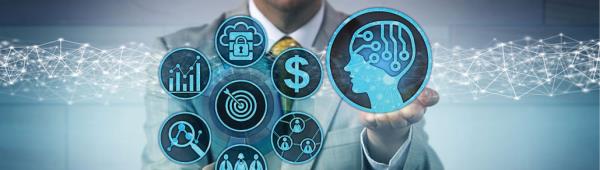
AI continues to plough through the IT sector, changing the networking world forever. What are the implications for IT teams; and is the governance where it needs to be?
Implementing AI into today’s networks requires a structured approach with several essential building blocks, encompassing technology, strategy, infrastructure, and governance to ensure the AI implementation achieves both business goals and complies with safety standards.
First steps
“AI can dramatically improve and accelerate network planning, operation and monetisation. Realising that potential cost-effectively and in a safe manner requires four building blocks to be in place,” says Abhishek Sandhir, Managing Director of Telecommunications for Sand Technologies.
First is a shared understanding of what success looks like and the problem the operator is trying to solve. Second up; the company needs a clear handle on its information journey: the data flows, exchanges, transformation and normalisation.
“Third is a dedication to compliance with GDPR, confidentiality and data sovereignty - which are important because they’re required for any data handling, and because this dedication can help build trust among those stakeholders who are still sceptical about AI,” shares Sandhir. “The fourth building block is a risk analysis of the decisions and activities that can be outsourced or automated versus left to human intervention, and the creation of any related guardrails that the enterprise deems necessary.”
AI systems require large volumes of high-quality, clean and diverse data. Implementing mechanisms to collect, integrate, and manage data from various network sources is essential. Data governance policies to manage data ownership, security, and privacy, while also ensuring data consistency and accuracy, are required. With this data comes the responsibility to ensure security; implementing encryption (both in-transit and at-rest) ensures that sensitive data remains secure throughout the AI processing lifecycle.
“Data is the cornerstone,” says Chris Gilmour, CTO at Axians UK. “AI systems often handle sensitive data, making security a top priority. Robust network infrastructure, data encryption, and access controls are essential to protect against unauthorised access and data breaches. Organisations must develop guidelines and frameworks to ensure that AI is used responsibly, avoiding biases, discrimination, and unintended consequences.”
Implementing AI at scale requires specialised skills, including data science, machine learning, model management, and AI ethics. Companies need to assess whether they have in-house expertise, particularly since AI will impact the day-to-day responsibilities of IT staff.
“Scaling AI across the network is a worthy goal, but every great journey begins with a first step,” opines Sandhir. “Before an enterprise can reach that target, they need to consider whether they have the capability in-house and/or with partners to understand, implement and manage a broad AI deployment. Scaling AI requires technical expertise of course, and partners bridge that gap effectively.”
Likewise, businesses should evaluate whether their current infrastructure can handle the workloads. High bandwidth and low latency networks are required to support data-intensive operations, especially when dealing with IoT devices or cloud-based AI applications.
Then comes the choice of AI algorithms and models. Using pre-trained models or open-source repositories can reduce development time and costs. Fine-tuning these models for specific tasks within the network can further optimise performance. For domain-specific use cases, custom AI models must be built and optimised for tasks like anomaly detection, predictive maintenance, or traffic routing.
Indeed, “the choice of AI algorithm is crucial. Deep learning, machine learning, and other techniques each have their strengths and weaknesses,” says Gilmour.
Rules and regulations
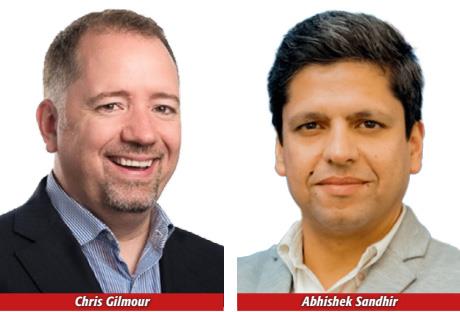
The UK’s regulatory framework for AI and data-driven technologies is relatively advanced, but there are concerns about whether it extends far enough.
Indeed, several gaps could be better addressed to ensure AI and data-driven technologies are used safely and responsibly. These include a comprehensive, AI-specific regulatory framework akin to the EU AI Act; the absence of a legally binding, overarching AI regulation leaves organizations without clear, standardised rules governing the development, deployment, and accountability of AI systems across all industries.
“While there are general principles governing AI ethics, more concrete rules and standards are needed to ensure that algorithms are fair, transparent, and accountable,” opines Gilmour. “Enforcement mechanisms should be strengthened to hold organisations accountable for any harmful or discriminatory AI applications.”
“As is expected when working with any fast-evolving technology, there are opportunities to improve,” shares Sandhir. “For example, more must be done to safeguard network vulnerabilities and mandate system resilience for major carriers, the recent Crowdstrike outage being just one recent example.”
This holds true particularly for high-risk areas like healthcare, law enforcement and critical infrastructure, where safeguards might be considered insufficient. In healthcare, an AI-driven diagnostic system can have life-or-death consequences, and regulations don’t sufficiently address the nuances of AI decision-making in these areas.
Bane or boon?
AI is both a boon and a potential bane for IT teams, depending on how it’s implemented and the context of use. The impact of AI on IT teams varies based on factors like job roles, organisational culture, and preparedness for AI-driven transformation.
On the boon side, AI can automate repetitive tasks, perform predictive maintenance, and detect system anomalies. With improved efficiency and productivity, AI systems can provide insights and recommendations to IT teams by analysing vast amounts of data; help diagnose and resolve issues quickly; and optimise resource allocation. Moreover, AI-powered cybersecurity tools can continuously monitor network traffic, user behaviour, and system logs to detect anomalies, while evolving in real-time to new and unknown threats.
“The potential benefits of AI are significant. By automating repetitive tasks, IT teams can free up valuable time to focus on more strategic initiatives,” confirms Gilmour. “AI can also help improve network performance, optimise resource allocation, and enhance security by detecting and responding to threats more effectively.”
In the bane column comes challenges with the skills gap, increased complexity in system management, and difficult integration into legacy system, which can require significant investment in time and resources. Beyond the security and ethical concerns, there also comes the risk of opaque decision-making; this lack of transparency can be problematic for IT teams, especially in high-stakes environments where explainability is crucial.
“It’s not hyperbole to say AI can, will and is revolutionising modern networks,” says Sandhir. “IT teams must therefore not only embrace the technology but lead the AI revolution. It is incumbent upon us all to create a culture where the UK is leading the way in AI and adopts a mindset of driving success through innovation, regardless of the level of complexity.”