05 May 2025
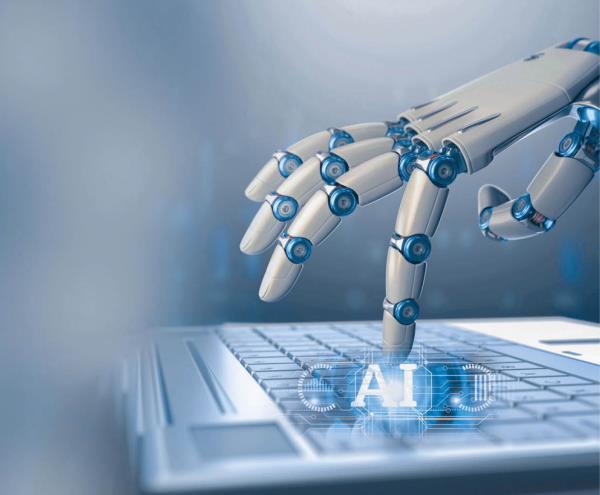
With AI seemingly taking the world by storm, is it having the predicted impact on network management teams, or has progress stalled?
As businesses pivot toward an increasingly digital landscape, the role of network management teams is on the cusp of a significant transformation.
“In 2025 and beyond, while core network environments (data centre, campus, WAN, cloud, hybrid cloud, and the emerging AI centre) remain largely consistent, their management is becoming significantly more intelligent and efficient thanks to AI/ML integration,” notes Praful Bhaidasna. Director of Product Management, Arista. “The AI Centre — purpose-built infrastructure designed to support high-throughput, low-latency, and compute-dense AI workloads — is rapidly becoming a distinct and critical environment for AI management. AI and ML are no longer good to have — they are becoming essential enablers of resilient, intelligent, and cost-efficient networks. As the pace of innovation continues to accelerate, AI won’t just enhance network management — it will redefine it.”
This shift, widely acknowledged as part of the fourth industrial revolution, will be largely driven by advancements in AI.
“Atos believes AI to be the fourth industrial revolution. It’s already transforming our work in network management, with more to come,” says Sean Wells, Private Sector Sales lead for Atos UK&I.
AI is expected to automate routine tasks, enable predictive maintenance, lower operational costs, and enhance network performance in real time. The implications are profound: network engineers will need to adapt to higher-skilled roles focused on strategic decision-making rather than mere manual operations.
According to Wells, “teams will shift to higher-skilled rather than manual tasks. AI will augment rather than replace human expertise. It’s a great time to be a skilled network engineer.”
Changing the game with predictive analytics
Across the UK, businesses are racing to digitally improve operations and user experience (UX) and with that comes sprawling, complex IT estates which increases the burden on IT teams. With the proliferation of remote work, cloud services, and complex Zero-Trust based network architectures, there are so many opportunities for blind spots that can lead to poor UX or IT outages if not addressed.
Nic Leszczynski, principal solutions engineer, Riverbed, explains that “AIOps can support stretched IT teams by autonomously monitoring networks. On top of traditional infrastructure-based monitoring data, IT Teams can leverage endpoint-based AI agents that collect a wealth of precious data. From application-aware network monitoring and real-time unified communication performance to Wi-Fi or Thunderbolt performance insights from the Intel chipsets and more, they effectively add more eyes on digital ecosystems to uncover blind spots and data gaps.”
Bhaidasna agrees that “assuming one has a good AI product and the right data being fed to it, the NetOps person’s job should be dramatically easier. A NetOps person would need operational awareness to interpret the insights provided by AI and translate them to mitigation steps while also possibly needing to integrate AI-driven network observability into existing, wider workflow automation tooling.”
The introduction of methods like Generative AI can enhance incident response capabilities, pinpointing critical concerns faster and putting the necessary expertise into action. Moreover, predictive AI not only identifies current issues but also highlights possible future risks, paving the way for proactive maintenance that can significantly reduce downtime and enhance service delivery.
According to Craig Smith, Technical Account Manager, Highlight, the most valuable implementations of AI for network teams are those that help improve productivity through faster identification and reduction of mean time to fix (MTTF).
“While there’s a lot of emphasis on preventive analysis - trying to stop issues before they occur – the current models can’t completely prevent failures. Instead, they help flag when the likelihood of failure is increasing, based on historical patterns,” notes Smith. “This becomes especially useful when scaled. For instance, if one type of device is showing signs of failure and the organisation has 1,000 similar devices, that insight becomes critical for proactive maintenance planning.”
“Beyond monitoring, they can also support by triaging issues quickly, making sure the right team is involved in the problem, and helping solve these issues faster leveraging multiple AI capabilities,” says Leszczynski. “Generative AI helps find the needle in a haystack by surfacing potential issues from the data and provide rich insights for investigations. Predictive AI analyses past and current data to spot trouble before it strikes. And Agentic AI can autonomously remediate issues. Ultimately, AIOps can support IT teams in managing and expanding networks required to fuel business growth.”
However, Smith believes that the adoption of AI to enhance the efficiency of network management workflows remains relatively slow, with most processes still largely manual.
“Currently, AI is primarily being used by individuals in back-office and engineering roles to support their personal productivity,” says Smith. “These applications are typically focused on routine tasks such as compliance checks, inventory analysis, and information summarisation, rather than being fully integrated into broader team workflows. Further, whilst it has been suggested that the potential OpEx reductions of 20-30% through AI-driven automation, the potential efficiency gains are around 30% for an engineer.”
The complexity of AI-driven automation
The integration of AI is not without risks. Smith raises concerns about unauthorized changes that may not be trackable, which can threaten operational integrity.
“The risk of data inadvertently crossing into another user’s domain is real and raises serious concerns - especially in sensitive sectors such as finance and healthcare - where data privacy and regulatory compliance are critical,” warns Smith.
The aspiration for ‘self-healing networks’ remains a challenge; current technologies, though promising, often fail to adapt network designs dynamically.
“Technologies like SD-WAN have made notable progress in bridging the gap, yet network designs remain largely static. The adoption of AI to proactively identify and recommend changes that could enhance network management continues to be slow and fragmented,” notes Smith.
While the promise of AI is enticing, implementing these innovations comes with notable challenges, especially in highly regulated sectors.
Leszczynski emphasizes that “organisations within heavily regulated industries like banking, insurance and healthcare can’t afford to have data that’s unaccounted for. Data caught up in blind spots and data gaps inevitably won’t be as secure as it should be. As regulations evolve to be more stringent around data protection and AI use, it’s even more crucial for organisations to have the right foundations and observability in place to ensure data quality and availability.”
“AI adoption is inevitable - it’s no longer a question of if, but how fast an organisation chooses to move.”
According to Smith, when it comes to enterprise and public sector environments where multiple users and customers have varying risk profiles, “a single implementation of AI-driven automation may not meet the requirements of all parties. The risk of data inadvertently crossing into another user’s domain is real and raises serious concerns - especially in sensitive sectors such as finance and healthcare - where data privacy and regulatory compliance are critical.”
As such, security considerations are critical when deploying AI-driven systems. Organizations must ensure that AI operates within a secure environment, ideally through self-hosted or privately managed cloud infrastructures.
“Using a Retrieval-Augmented Generation (RAG) model is essential to feed AI agents with the specific knowledge required to answer both internal queries and customer questions about the services being managed,” adds Smith. “Additionally, it’s critical that any system prompts used within AI agents are carefully crafted to ensure they are relevant, ensuring the AI-agent behaves and communicates in a way that resonates with the customer. They must also be trusted, building confidence in the AI’s ability to understand and respond to customer needs accurately. Most importantly, ethical considerations must be at the forefront, addressing potential bias and promoting the responsible, fair use of AI technologies.”
“Integrating AI-driven automation into network operations brings tremendous efficiency and intelligence. And in regulated environments like enterprise or the public sector, it’s a great chance to thoughtfully navigate things like security and compliance — turning potential challenges into opportunities to build even stronger, more resilient systems,” says Bhaidasna.
By adhering to these principles, organizations can effectively leverage AI while safeguarding operational security and the trust of users.
An AI-enabled future
As AI adoption accelerates, change management and workforce upskilling are essential for successful integration. The decisive factor in the speed of AI deployment will depend on organizations’ willingness to embrace an inclusive approach.
“AI adoption is inevitable - it’s no longer a question of if, but how fast an organisation chooses to move,” asserts Smith. “If an organisation insists that adoption must be entirely smooth, strictly follow approved change control processes, and be managed by a small, centralised team, then progress will likely be slow, taking anywhere from 6-12 months. For network management teams, the urgency is growing. Those not leveraging AI within the next 4-6 months risk falling behind, and senior management may begin to question why AI is not yet part of the service offering.”
Effective adoption, however, requires a cultural change within organizations.
“To streamline AI deployments and drive the most value from them, AI teams help by training people on how to interact correctly with AI tools,” recommends Leszczynski. “Educating IT teams about the challenges caused by blind spots and data siloes… is crucial for uncovering them.”
Smith believes that the central conundrum surrounding AI in network operations is whether it will augment human roles or replace them – “I see AI primarily as an augmentation tool - an intelligent assistant that enables network operations teams to gain faster insights into customer networks, ultimately improving incident management and reporting. The only area where I foresee full automation is in customer-facing AI assistants, such as those that handle basic queries. These include providing service details, accessing knowledge base information, or offering general help and support. In these cases, AI can efficiently manage routine interactions, freeing up human resources for more complex tasks.”
“AI in networking isn’t about job loss — it’s about job evolution,” concurs Bhaidasna. “Engineers and IT teams will shift from being device operators to system orchestrators and experience managers.”
“For AI to succeed, it requires an organisation-wide cultural change and AI teams help by training people on how to interact correctly with AI tools to smooth learning and development,” adds Leszczynski. “Similarly, AI teams can also support in ensuring the right environment for AI, for instance by educating IT teams about the challenges caused by blind spots and data siloes which are often a consequence of sprawling, complex IT estates and the need for AIOps and observability tools to uncover them.”
Ultimately, the roll-out of AI in enterprise network management teams is not just about technology; it’s fundamentally about people. As teams evolve, embracing these innovations can lead to unprecedented improvements in operational efficiency and service delivery. It is a transformative period for network engineers, who will find themselves better equipped to handle the complexities of modern network environments. Embracing AI will not only enrich their roles but will redefine the landscape of enterprise network management in the UK.
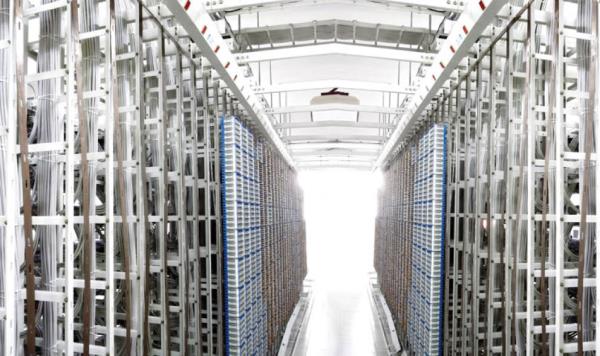