07 August 2020
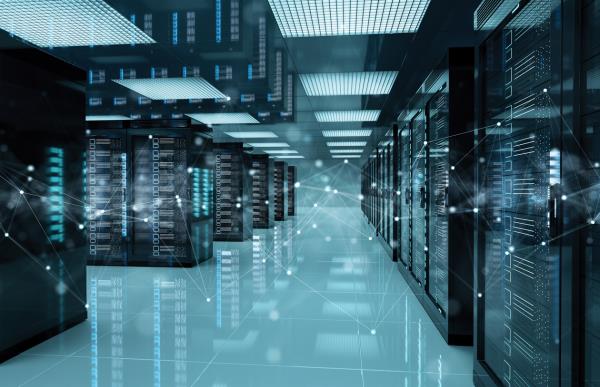
Admit it, the first time some of you heard of ‘Artificial Intelligence’ was following the release of A.I. - the eponymous 2001 motion picture, developed by Stanley Kubrick in the 70s and directed by Steven Spielberg decades later.
It was to be the former’s first foray into the science-fiction genre since his 1968 sci-fi classic 2001: A Space Odyssey, a prescient allegory about how destructive artificial intelligence can be when cruelly misused. The point is visionaries like the Hollywood creatives foresaw how useful it could be to humankind before most people reading this were born.
Now, fast-forward to Gartner’s 2019 CIO Survey, which highlights the fact that number of enterprises implementing AI tripled in the past year. However, Gartner also claims that more than 30% of data centres that don’t deploy AI and machine learning won’t be operationally and economically feasible by 2020.
Still, before even understanding the potential use cases for data centres, John Yardley, CEO, Threads Software, says it’s prudent to first understand what AI means. “It was, of course, Alan Turing who originally coined the term machine (or artificial) intelligence to define the machine behaviour that is indistinguishable from that of human,” he adds. “So, when you ask Siri lots of questions and it gives you the answers you would expect, you might regard that as artificial intelligence. In fact, Siri may be simply doing a web search which is just looking up millions of web resources. To the user, that is just as intelligent as an application that is modelling the human brain. In fact, it is the speech recognition that is the AI rather than web search, but the user would not necessarily perceive it that way.” Yardley says that “it is also important to realise that the human brain is a computer” and that “there is technically no reason” why its behaviour could not be modelled in silicon - together with all the emotions we perceive as “human”.
Robots can carry out the vast majority of functions we mere mortals have always carried out ourselves and Yardley sees a lot of benefit in that.
“Computers do not get tired, want pay rises, get sick, or throw tantrums,” he continues. “AI is simply another form of automation, just like the Jacquard machine (a device fitted to a loom that simplifies the process of manufacturing textiles with complex patterns) was. It displaces humans, but the replaced humans can then be deployed to do things computers cannot yet do. Humans are not good at repetitive boring tasks.”
Yardley adds that in the data centre, there are many such tasks. “Filing responses, looking up records, pre-empting questions and so on,” he continues. “What AI can do is find connections that humans cannot, or at least, much more quickly, such as establishing that certain sorts of customer have certain sorts of questions. Huge amounts of information is conveyed in people’s voices that might not be recognised by a human, but detectable by a computer.”
For Peter Ruffley, chairman at data specialist Zizo, “the IT industry is doing itself no favours” by promising the earth with emerging technologies, without having the ability to fully deliver them. “See Hadoop’s story with big data as an example and look where that is now,” he says. “There is also a growing need to dispel some of the myths surrounding the capabilities of AI and data-led applications, which often sit within the c-suite, that investment will give them the equivalent of the ship’s computer from Star Trek, or the answer to the question ‘how can I grow the business?’ As part of any AI strategy, it’s imperative that businesses, from the board down, have a true understanding of the use cases of AI and where the value lies.” Ruffley adds that if there is a clear business need and an outcome in mind then AI can be the right tool. “But it won’t do everything for you,” he warns. “The bulk of the work still has to be done somewhere, either in the machine learning or data preparation phase.”
Understanding how something works is clearly the most important thing, but then you have to understand “what the whole strategic vision is and look at where value can be delivered and how a return on investment (ROI) is achieved”. That’s Ruffley’s view and he says what needs to happen is for data centre providers to work towards educating customers on what can be done to get quick wins.
“Additionally, sustainability is riding high on the business agenda and this is something providers need to take into consideration,” he adds. “How can the infrastructure needed for emerging technologies work better? Perhaps it’s with sharing data between the industry and working together to analyse it. In these cases, maybe the whole is greater than the sum of its parts. The hard bit is going to be convincing people to relinquish control of their data. Can the industry move the conversation on from being purely technical and around how much power and kilowatts are being used to how is this helping our social corporate responsibility/our green credentials?”
Ruffley also highlights a number of innovations already happening, where lessons can be learnt. In the Nordics, for example, there are those who are building carbon neutral data centres, which are completely air cooled, with the use of sustainable power cooling through solar. “The cooling also comes through the building by basically opening the windows,” he adds. “There are also water cool data centres out there under the ocean.”
Jonathan Martinez, commercial control systems manager at data centre cooling solutions provider’ Airedale says there are a number of benefits arising from the use of AI/machine learning within a data centre environment, which Airedale is developing within the ACIS AI framework. “One of the big ones being the ability to autonomously optimise the HVAC system to ensure it is always running at peak efficiency,” he says. “Often cooling systems are ‘set and forget’ once commissioned, however variables such as data centre IT load and outside ambient temperature can vary throughout the course of the year. The cooling system in its commissioned state is sized to deal with these fluctuations, however at peak load may not be operating at peak efficiency.”
Martinez says a machine learning algorithm can learn the patterns associated with these variables and determine over time what changes it can make to optimise the efficiency of the plant during the pattern cycle. “If the system can predict when these peaks will happen, then it can take action prior to these peaks to ensure the system is best armed to deal with the spike in the most efficient way,” he adds. “This could be adjusting chilled water setpoint, altering airflow, or reducing pump setpoints for example. In short, an AI system in theory could make constant minute adjustments to the system throughout the year to improve efficiency based on pattern recognition, far better than a human could.”
Then, of course, there’s that old debate of AI ready vs. AI reality and Ruffley questions why with IoT, many organisations are chasing the mythical concept of ‘let’s have every device under management’. He continues: “But why? What’s the real benefit of doing that? All they are doing is creating an overwhelming amount of low value data. They are expecting data warehouses to store a massive amount of data. If a business keeps data from a device that shows it pinged every 30 seconds rather than a minute, then that’s just keeping data for the sake of it. There’s no strategy there. The ‘everyone store everything’ mentality needs to change.”
Indeed, he says one of the main barriers to implementing AI is the challenges in the availability and preparing of data. He claims a business cannot become data-driven, if it doesn’t understand the information it has and the concept of ‘garbage in, garbage out’ is especially true when it comes to the data used for AI.
Ruffley predicts that over the coming years, the world will see “a tremendous investment in large scale and high-performance computing (HPC) being installed within organisations to support data analytics and AI.
“At the same time, there will be an onus on data centre providers to be able to provide these systems without necessarily understanding the infrastructure that’s required to deliver them or the software or business output needed to get value from them,” he says. “We saw this in the realm of big data, when everyone tried to swing together some kind of big data solution and it was very easy to just say we’ll use Hadoop to build this giant system. If we’re not careful, the same could happen with AI. There’s been a lot of conversations about the fact that if we were to peel back the layers of many AI solutions, we’ll find that there is still a lot of people investing a lot of hard work into them, so when it comes to automating processes, we aren’t quite in that space yet. AI solutions are currently very resource heavy.”
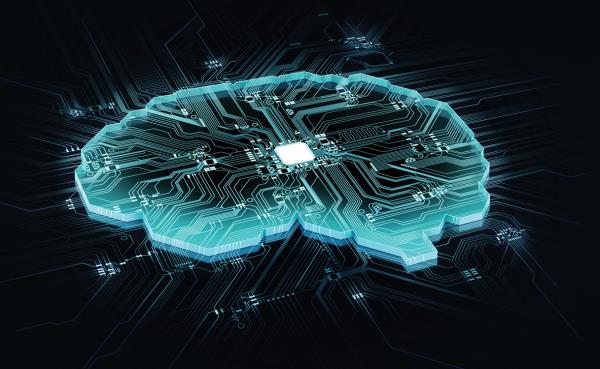
Looking to the future, will real life resemble a Hollywood sci-fi movie in that everything will be run by AI, or will we always need humans on standby?
He says “there’s no denying that the majority of data centres” are now being asked how they provide AI solutions and how they can assist organisations on their AI journey. “Whilst organisations might assume that data centres will have everything to do with AI tied up. Is this really the case?,” Ruffley continues. “Yes, there is a realisation of the benefits of AI, but actually how it is best implemented, and by who, to get the right results, hasn’t been fully decided.”
If you know anything about AI, then you’ll have heard of the ‘black box problem’, because like the human brain, it is hard to understand from the outside. The black box is used for decision-making, often based on machine learning over big data, mapping a user’s features into a class predicting the behavioural traits of individuals, without exposing the reasons why. As you can imagine, it can be problematic not only for its lack of transparency, but also for possible biases inherited by the algorithms from human prejudices and collection artifacts hidden in the training data. That’s because they can lead to unfair, unexpected or wrong decisions.
Ted Kwartler, VP, Trusted AI at enterprise AI specialist DataRobot, says AI in the datacentre faces the same challenges as AI in any other industry or application. “When the ‘black box problem” in AI is raised, in response, some may focus on the evolving techniques that allow us to probe, stress-test, or challenge the opaquest of our models,” he says. “Others may point to the fact that ‘explainability’ should inform model selection. Depending on the use case, some modelling techniques support transparency far better than others without sacrificing performance. There’s no such thing in machine learning as one-size-fits-all.”
However, Kwartler warns of “a bigger and actually even more vital question lurking underneath the ‘black box problem’.” He says: “Why is it so important to explain a model’s decision? If a model performs perfectly – if its decision-making is flawless – we wouldn’t worry so much about explaining how it works, besides learning from it. However, no such model exists in real life. So, when we’re talking about an algorithm making a decision of high-impact on a person’s well-being, for example, and maybe getting it wrong, we need to be able to do more than just explain a bad prediction. We want to be able to prevent it.”
Perceived wisdom would dictate that the underlying issue is how to establish trust in a model’s decisions. That’s because AI models from development to production need operational guardrails to ensure trustworthy behaviour, adds Kwartler .”In model training, building trust first means interrogating the data: its integrity, diversity, appropriateness and quality,” he continues. “Model selection is then often a negotiation of trade-offs, balancing accuracy, speed and explainability across a variety of suitable techniques. And finally, in production, proper governance requires humility: the ability to recognise how confident a prediction is and if there are quantifiable reasons to be unsure of it. If so, a human-in- or human-over-the-loop should be empowered to intervene and guarantee that a safe decision is made. Explainability is possible in AI, but achieving it is not the finish-line for actually building a model you can trust.”
Yardley again invokes World War II when he explains that the German Enigma machine – Turing as we know cracked its codes – was a good example of a black box in that it is unlikely any of the operators knew or cared how it worked. “In contrast, the data encryption standard (DES) is not a black box - the way it works is public,” he adds. The strength of the DES is in the algorithm itself, not in it being secret.”
So, is the ‘black box problem’ a serious one? Yardley certainly thinks so. “Not because we need to know how AI works but because a black box approach discourages developers from understanding the problem they are solving,” he says .”Take neural network approaches to speech recognition. These work well at recognising speech but the developers have no idea why they work - that is, the developers know nothing about acoustics, semantics, linguistics, and so on, and nor do they need to know. They tend to assume that the more computing power is applied, the better the result. However, relying solely on historic examples to predict future performance usually results in hitting a dead end and having no idea what to do.”
“With any system that we don’t fully understand, we cannot predict its behaviour,” he continues. That could have legal and even potentially more serious implications.”
Martinez agrees that the black box issue is a valid concern. “Techniques such as linear regression, by design, are more interpretable compared to techniques such as deep learning,” he says. “However, interpreting deep learning models aka ‘explainable AI’ is a hot topic of research and there are multiple ways to mitigate the issue. There are multiple machine learning toolboxes to extract additional information from machine learning (ML) models in general, Google have a decent suite of these already. One of the design requirements of the AI solutions we are developing at Airedale, is for the system to be fully interpretable in order to avoid the ‘black box problem’.”
Looking ahead to the future, will real life resemble a Hollywood sci-fi movie in that everything will be run by AI, or will we always need human hands on standby?
Nevertheless, Ruffley warns, that lots of data centres “jumped in headfirst” with the explosion of big data and didn’t come out with any tangible results. “If we’re not careful, AI could just become another IT bubble,” he says.
Nevertheless, Ruffley says “there is still time to turn things around”, because as we move into a world of ever-increasing data volumes, we are constantly searching for the value hidden within low value data that is being produced by IoT, smartphone apps and at the edge. “As the global costs of energy rise, and the numbers of HPC clusters powering AI to drive our next generation technologies increase, new technologies have to be found that lower the cost of running the data centre, beyond standard air cooling,” he continues. “It’s great to see people thinking outside of the box on this with, with submerged HPC systems and full, naturally aerated data centres, but more will have to be done (and fast) to meet up with global data growth. The appetite for AI is undoubtedly there but for it to be able to be deployed at scale and for enterprises to see real value, ROI and new business opportunities from it, data centres need to move the conversation on, work together and individually utilise AI in the best way possible or risk losing out to the competition.”
As far as Yardley is concerned, “If we can clone a human brain, it will act indistinguishably from the human’s brain it was cloned from”. He says: “That may take another 100 years, but if we don’t self- destruct, it will happen. That of course raises many questions about the future of the human race.”
That’s a much deeper discussion for another time and place. It’s also a good stimulus for another Hollywood blockbuster.