06 May 2025
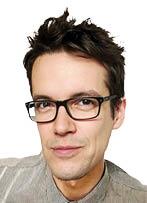
Bernie Malouin, VP, Design, Process and Technology Engineering, JetCool, A Flex Company
The relentless growth of AI is pushing the boundaries of computing power, and at the heart of these advances lies a quiet but critical struggle: keeping the machines cool.
Take Meta’s Llama 3 405B, for instance. Over the course of 54 days, Meta’s AI infrastructure experienced daily GPU failures. Meta experienced nearly eight unexpected failures a day over this training run, and 58.7% were attributed to GPU failures. Compared with other components (especially CPUs and system memory), it’s clear that AI training puts distinctively intense pressure on the GPUs and as a result, they fail.
GPU reliability
When a GPU fails in an AI training cluster, the ripple effects are both immediate and expensive. Industry experts estimate that GPU downtime costs can range from $500-2,000 per hour per node. Beyond direct replacement costs, the operational toll is considerable, including training delays, lost productivity, escalating costs, and efficiency loss: a 1% drop in cluster utilisation across a deployment of 1,000 GPUs (distributed across 125 servers costing $350,000 each) would result in an annual financial impact of approximately $437,500.
These numbers paint a stark picture: GPU failures are not just technical inconveniences — they’re financial liabilities.
Temperature fluctuations play a pivotal role in GPU reliability. Meta’s experience with performance variations linked to mid-day heat is far from unique. Across data centres, diurnal temperature patterns drive operational challenges. Average daily temperature variations can degrade server performance, while during summer peaks, increased temperature deltas lead to increased fan power consumption. In dense GPU deployments, the problem compounds.
Even with state-of-the-art fans, maintaining optimal temperatures is a formidable challenge.
Traditional air cooling systems, though ubiquitous, struggle to keep pace with modern GPUs. Thermal imaging reveals why: non-uniform heat distributions create hot spots that air simply cannot target effectively. The consequences include localised overheating, thermal stress, and inefficiency.
Extending GPU lifespan
Temperature and reliability are closely intertwined. Research shows that every 10°C reduction in operating temperature doubles a semiconductor’s lifespan.
Using direct-to-chip liquid cooling using microjets, GPU temperatures can lower by 30°C compared to air cooling, translating to an 8x improvement in theoretical lifespan. This is where microconvective liquid cooling technology comes into play. By addressing heat at its source, this type of microjet impingement cooling offers a transformative solution of targeted cooling, consistent thermal management, and scalability for AI workloads. For organisations heavily invested in AI infrastructure, these gains are not just technical — they’re strategic.
Comprehensive testing of NVIDIA H100 GPUs highlights the significant impact of direct-to-chip liquid cooling with microjets. When evaluating thermal resistance — a critical metric for heat transfer efficiency — air cooling systems lag, with a thermal resistance of approximately 0.122 °C/W compared to significantly lower values achieved by advanced liquid cooling solutions.
Beyond thermal resistance, large-scale AI deployments stand to benefit significantly from reduced energy costs. In a fleet of 2,000 GPUs valued at $33 million, traditional cooling methods can drive annual power costs of around $2 million. Direct-to-chip liquid cooling with microjets provides a more efficient alternative, cutting cooling energy consumption by up to 30% over conventional methods.
By reducing facility cooling demands, this approach lowers operational expenditures, enables scalable AI growth, and minimises the environmental footprint of high-performance workloads.
Even under challenging conditions — such as operation with a 60°C PG25 coolant — advanced liquid cooling ensures GPUs remain safely below throttling limits. This guarantees stable performance in demanding environments, allowing data centres to maintain efficiency while meeting the increasing computational requirements of AI-driven workloads.
A future-ready cooling solution
As AI workloads continue to grow, rethinking cooling strategies is critical for maximising GPU performance and longevity. By transitioning from air to liquid cooling, organisations can ensure their AI infrastructure remains reliable and costeffective, meeting the increasing demands of high-performance computing while reducing operational risk and environmental impact.